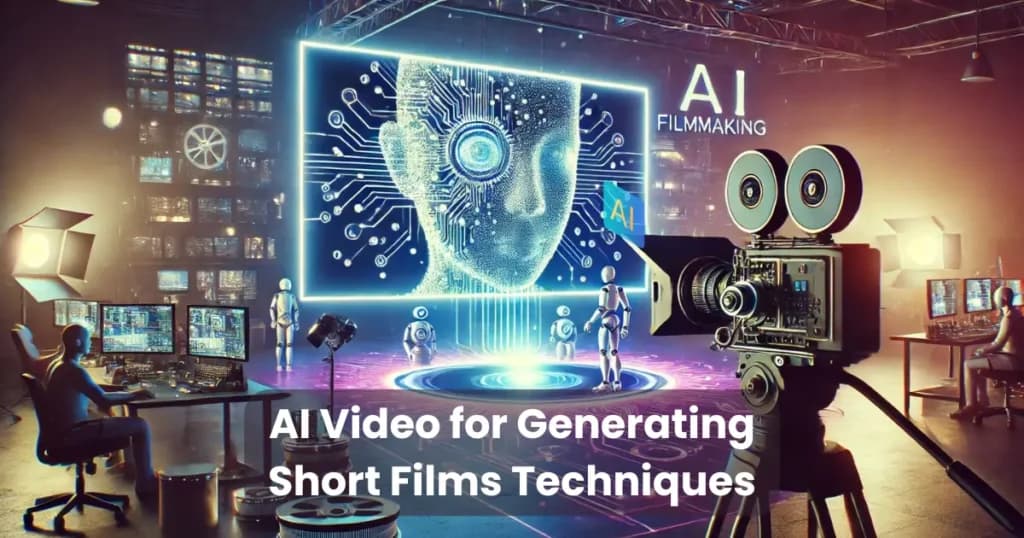
Contents
In today’s fast-evolving digital landscape, the process of text-to-video AI explained has become a topic of growing interest among content creators, marketers, and educators alike. This revolutionary advancement allows machines to generate compelling video content directly from written text, eliminating the need for complex editing tools or technical expertise.
As artificial intelligence continues to improve, so too does its ability to interpret language, visualize scenes, and produce realistic motion. Moreover, this technology not only saves time but also empowers creators to bring their ideas to life with minimal effort. In this article, we’ll take a comprehensive look at how text is converted into video, what technologies are involved, and why this process is set to transform the future of multimedia content.
To fully grasp the process of text-to-video AI explained, it is important to understand the core concept behind this innovative technology. At its essence, text-to-video AI is a system designed to transform written language into video content using artificial intelligence and machine learning techniques.
Unlike traditional video production, which often requires a team of editors, animators, and voiceover artists, this technology automates the entire workflow. By analyzing the input text, the AI can detect the tone, context, and key visual elements described. Afterward, it generates corresponding scenes, characters, animations, and even voiceovers without manual input.
Furthermore, these systems are often powered by a combination of Natural Language Processing (NLP), computer vision, and generative models like GANs (Generative Adversarial Networks) or diffusion-based networks. These models work in harmony to interpret semantics and render visuals that match the narrative’s intent.
Because of these capabilities, text-to-video AI is not only efficient but also accessible to individuals with no background in animation or video editing. As a result, it is increasingly being adopted across industries ranging from education and advertising to gaming and digital journalism.
To better understand the process of text-to-video AI explained, it’s essential to break down the steps involved in transforming written text into fully rendered video content. Each phase in the pipeline contributes to the system’s ability to generate visuals that accurately reflect the narrative.
First and foremost, the user inputs written content into the platform. Then, the AI utilizes advanced Natural Language Processing (NLP) to interpret the meaning, tone, and context of the text. Key entities, settings, actions, and emotions are extracted automatically to lay the foundation for scene generation.
Once the text is analyzed, it is segmented into a scene-by-scene structure. During this stage, the AI identifies how the narrative should be visually divided. For instance, each sentence or paragraph might correspond to a new setting, a shift in dialogue, or a character interaction. Additionally, camera angles, moods, and transitions are pre-configured based on context.
Afterward, the system either generates or retrieves visual assets needed for each scene. These include backgrounds, characters, props, and special effects. Assets are matched with the text through AI training on vast image and video databases, ensuring relevance and coherence.
Next, the generated elements are placed into animated environments. Characters are positioned according to the script, while movements and actions are automatically choreographed. Meanwhile, camera motions such as zooms or pans are added to enhance storytelling.
Following scene composition, voiceovers and audio effects are incorporated. Text-to-speech engines generate natural-sounding dialogue in a variety of languages and accents. Additionally, background music and environmental sounds are layered in to improve immersion and emotional resonance.
Lastly, the video is compiled and rendered into its final form. During rendering, elements like lighting, timing, and resolution are fine-tuned for quality. The completed video is then ready for download, sharing, or embedding across platforms.
Now that the process of text-to-video AI explained step by step is clearer, it’s crucial to explore the technologies that make this transformation possible. Each phase of the pipeline relies on a specific set of AI-driven tools that work together to interpret, generate, and refine video content from textual descriptions.
To begin with, NLP plays a foundational role. It allows the system to understand and interpret human language with nuance. Through techniques like sentiment analysis, entity recognition, and semantic parsing, the AI can determine not just what is said—but how it is meant.
At the core of text interpretation are transformer-based models such as GPT (Generative Pre-trained Transformer) and BERT (Bidirectional Encoder Representations from Transformers). These models help the system grasp context, predict meanings, and generate coherent scene instructions from raw text.
Next, computer vision is used to align text-based ideas with visual representations. With the help of image recognition and spatial understanding, the system determines where characters, objects, and environments should appear in the frame.
In addition, GANs are used to generate high-quality visual assets. One network creates images or animations, while another evaluates their realism. This back-and-forth process results in refined, life-like visuals tailored to the input script.
Furthermore, diffusion models—commonly used in tools like Stable Diffusion—assist in producing photorealistic frames. These models begin with random noise and gradually refine the image through a guided, AI-driven process, which is especially effective for abstract or artistic visual requests.
Last but not least, modern text-to-speech (TTS) technologies enable realistic voiceovers. These engines can simulate human-like intonation, emotion, and timing, ensuring the spoken word aligns perfectly with the animated visuals.
With the process of text-to-video AI explained and the underlying technologies detailed, it’s time to explore how this innovation is already impacting a variety of industries. From education to marketing, the possibilities are both diverse and dynamic.
First and foremost, educators are using text-to-video AI to create engaging learning materials. Lesson plans, lectures, and tutorials can now be transformed into interactive videos, making content more accessible and digestible for students. Furthermore, language support and automated narration allow for global reach.
In the business world, marketers benefit greatly from the speed and flexibility of text-to-video tools. Product descriptions, brand stories, and promotional messages are easily turned into eye-catching videos. As a result, campaigns can be launched faster and at lower costs than with traditional video production.
Meanwhile, content creators—including YouTubers and social media influencers are adopting text-to-video AI to streamline their production pipelines. Scripts can be turned into animated shorts, explainer videos, or character-driven scenes without the need for extensive software knowledge.
In digital journalism, newsrooms are using the technology to visualize breaking stories and reports. Articles can be enhanced with visual summaries, making them more engaging for audiences who prefer video over text.
Additionally, corporations are leveraging AI-generated videos for onboarding, compliance training, and internal communication. Training materials that once took weeks to produce can now be delivered in hours—customized for different teams or roles.
Lastly, healthcare organizations are applying text-to-video AI to create public awareness content. Whether it’s explaining symptoms, preventive measures, or treatment options, these videos help bridge the gap between medical jargon and patient understanding.
Even with the process of text-to-video AI explained thoroughly, it’s important to acknowledge the challenges that still limit the technology’s full potential. While rapid advancements have been made, several technical and creative obstacles remain in the current pipeline.
To start, natural language is often ambiguous. The same sentence can have multiple meanings depending on context, tone, or cultural nuance. Because of this, AI may misinterpret the intended message, leading to visual outputs that feel off-topic or misleading.
Moreover, maintaining visual consistency across multiple scenes is a significant hurdle. Characters may change appearance between shots, or background elements may shift unintentionally. This disrupts narrative flow and reduces the professionalism of the final output.
Although the AI is capable of producing impressive results, it still struggles with creative nuance. Subtle visual cues, artistic flair, or abstract ideas are difficult for machines to grasp without explicit instruction. Consequently, creative professionals may find the results lacking depth.
Another common challenge involves audio synchronization. While text-to-speech systems can generate lifelike voices, aligning those voices with animated lip movements often results in unnatural or mismatched timing—particularly in character-driven scenes.
Furthermore, rendering high-quality videos remains time-consuming and computationally intensive. Despite automation, delays may still occur, especially for longer or more complex scripts. Additionally, real-time generation at scale is still not feasible for many systems.
Lastly, AI-generated videos can reflect biases present in training data. This raises ethical concerns, particularly when content relates to sensitive topics like race, gender, or politics. If not properly managed, such biases can undermine the credibility and inclusivity of the content.
With the process of text-to-video AI explained in detail and its current limitations outlined, attention now turns toward the future. As technology evolves at an unprecedented rate, the potential for text-to-video AI is both vast and inspiring.
To begin with, future systems are expected to become increasingly context-aware. By incorporating user preferences, previous behavior, and real-time data, AI-generated videos will feel more personalized and relevant than ever before.
In addition, advancements in rendering engines and neural networks will continue to push the boundaries of realism. Photorealistic characters, seamless animation, and accurate lip-syncing will likely become standard features—even in consumer-grade platforms.
Soon, real-time text-to-video generation could become a reality. As computing power increases and algorithms become more efficient, the time between script input and final output will shrink drastically, making it feasible for live applications like interactive storytelling or dynamic marketing.
Moreover, integration with other AI technologies—such as chatbots, virtual assistants, and AR/VR platforms—will allow text-to-video AI to serve as a key component in immersive digital experiences. For example, customer support could evolve into animated, voice-guided interactions created instantly from a query.
Another exciting development is increased accessibility. As interfaces become more intuitive, users with no technical or design background will be able to produce professional-grade videos. This democratization of content creation could empower educators, journalists, entrepreneurs, and creatives across the globe.
At the same time, the industry will likely see a stronger focus on ethics and accountability. Built-in content moderation, fairness algorithms, and transparent AI models will become essential in ensuring the responsible use of text-to-video technologies.
In summary, with the process of text-to-video AI explained from start to finish, it becomes evident that this technology is more than a futuristic novelty it’s a transformative tool reshaping how content is created and consumed. From education to entertainment, and from marketing to healthcare, its applications are both expansive and impactful.
While there are still technical and ethical challenges to overcome, continuous advancements in natural language processing, computer vision, and generative AI are steadily enhancing the system’s capabilities. As these technologies mature, we can expect even more accurate, creative, and personalized video outputs all generated with just a few lines of text.
Ultimately, the process of text-to-video AI represents a pivotal evolution in digital storytelling. Whether you’re a brand, educator, or content creator, now is the time to explore how this innovation can elevate your message, streamline production, and engage your audience in new and compelling ways.
© 2024 LeyLine